Yukiya Saito
NP3M fellow, University of Notre Dame and Facility for Rare Isotope Beams, Michigan State University
E-mail: ysaito@nd.edu ; saitoy@frib.msu.edu
CV Publications List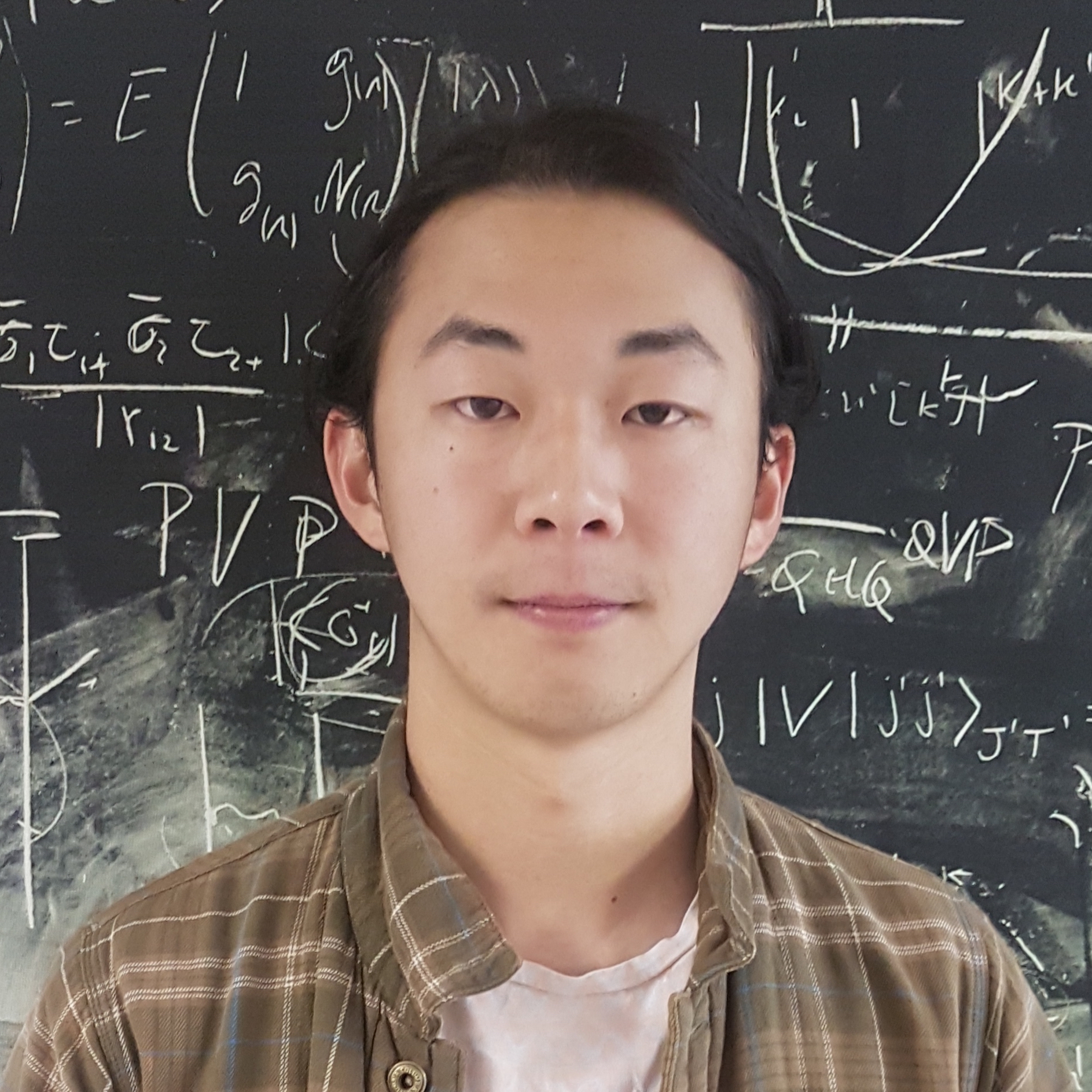
Research Interests
Heavy element nucleosynthesis in the rapid neutron capture process (r-process), Bayesian Statistics, machine learning applications in theoretical calculations and experimental data analysis, and nuclear density functional theory.
About Yukiya Saito
I am a nuclear astrophysicist, whose primary goal is to understand the origin of heavy elements in the Universe, from the perspective of microscopic nuclear physics. The primary tool that I use for my studies is nuclear reaction network calculations, which compute the evolution of nuclear abundances in astrophysical events such as neutron star mergers and some types of core-collapse supernovae, in the case of the r-process nucleosynthesis.
In these calculations, in addition to realistic astrophysical simulations, the nuclear physics inputs, such as nuclear masses, beta-decay rates, neutron capture rates, fission properties, and many other quantities, play an essential role. While a large amount of effort has been devoted to measuring these quantities experimentally, theoretical descriptions are also crucial, especially in the neutron-rich region.
My research involves studying the effect of nuclear physics input on these calculations, as well as developing new methods to quantify the uncertainties and to improve the descriptions of the properties of neutron-rich nuclei. I use a variety of techniques in addition to nuclear reaction network calculation, such as Bayesian statistics, machine learning, and nuclear density functional theory, to perform these studies.
I am also interested in the intersection of theoretical and experimental nuclear physics, since I previously worked on experimental nuclear physics, where I studied the properties of neutron-rich nuclei with gamma-ray spectroscopy.
Some of my recent research highlights include:
- Uncertainty quantification of nuclear masses with ensemble Bayesian model averaging
We developed a new method to quantify the uncertainties of nuclear masses using a Bayesian technique for the mass models that are commonly used in the r-process studies. - Emulation of the calculations of final r-process abundance patterns with a neural network
We developed a new method to emulate the calculations of final r-process abundance patterns with a neural network, which can be used to obtain the r-process abundance patterns for different nuclear physics inputs.